K#5. The only Data Analysis framework you'll ever need
How to conduct deep dives for behavioural or impact analysis
Heyyy 👋
Welcome back to K’s DataLadder ✨! Each week I share a story from my life as a Tech Data Scientist to help you level up your career.
We’ve grown to an amazing 753-strong community of curious and driven data whizz. Thanks to each of you for tuning in ❤️
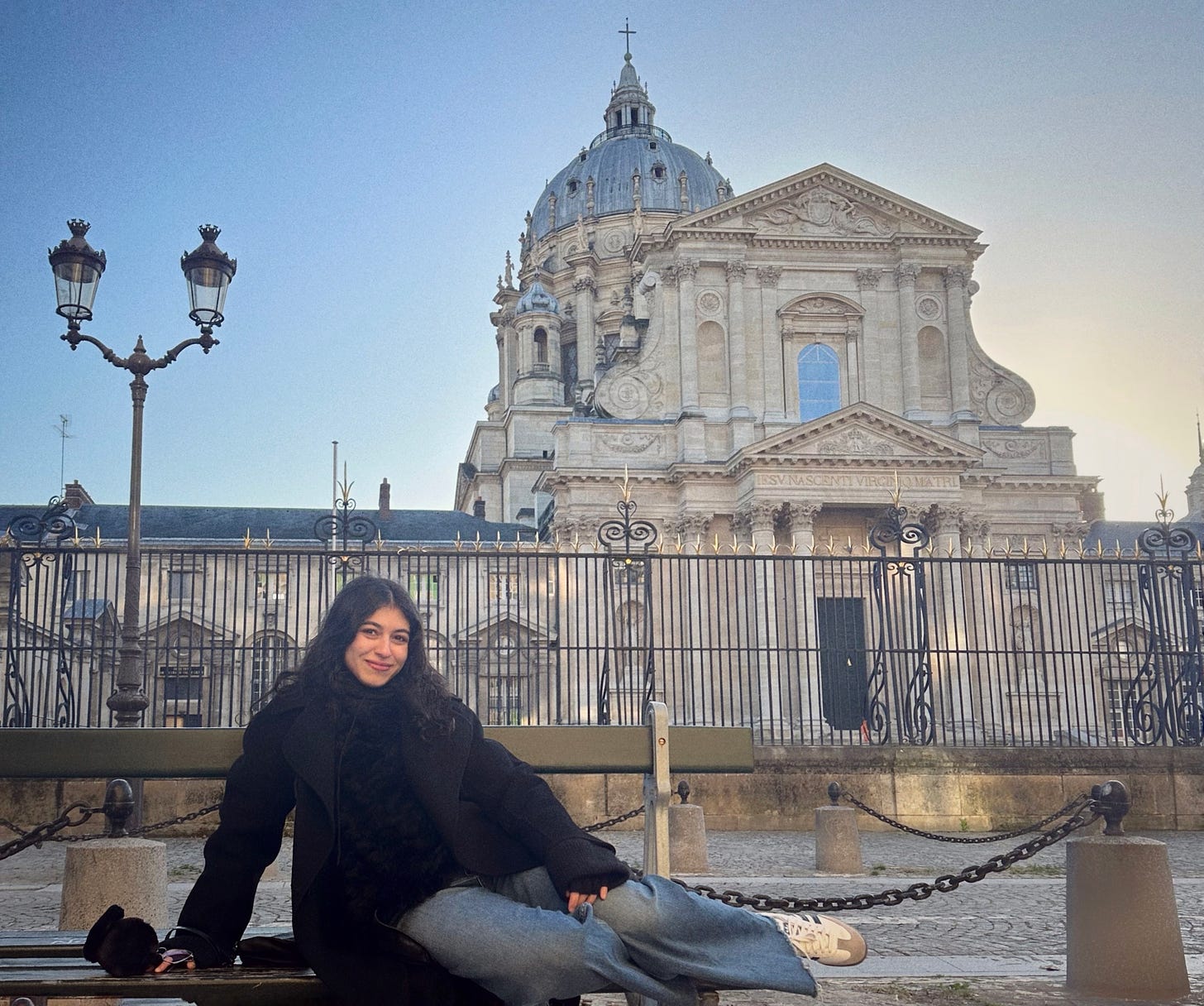
In this week’s edition, I want to share with you a bulletproof framework that you can use to conduct data analysis. I work in a product-centric company so a big part of our job as data scientists is to bring answers to questions no one has answers to.
Estimated Reading Time: 5 minutes
But first, if you haven’t done that already, you can:
Agenda
This week’s story
Why you should care
How to approach deep dives
This week’s story
Spotify has recently launched a game-changing feature that I’m so excited about: Music Videos.
I work with the team responsible for the launch, so as a data scientist, it becomes one of my responsibilities to assess the overall impact of this release on our platforms. I joined Spotify because I’m a violinist and I love music so getting to work on music-related topics is such a perk and I’m grateful for it.
Due to confidentiality reasons, I can’t get into more details, but you get the gist.
So like I said, I’m conducting a deep dive analysis on the topic, and while I was brainstorming and planning the overall research plan, it made me realise I had gotten rusty on the whole deep dive approach.
How do you actually approach such foundational behavioral research?
That’s the question I asked my colleagues in our biweekly data science forum – a meeting where we discuss all things data or job-related.
My team shared super insightful inputs and I’ve gathered these into a guide, which I’m thrilled to share with you today.
I hope it’ll help you become more efficient in your data analysis approach.
But first, why should you care?
Deep dives, or foundational data analysis, are vital in product-driven companies like ours.
It’s a fundamental part of the job because ultimately, we’re here to help further business goals. Whenever a product company releases new features, it becomes our duty, as data scientists to:
Understand user experience
Analyse the impact on our metrics
Refine our strategies for future developments
Essentially, we need to figure out if it was a good bet. We do this by:
Performing A/B tests on small samples before releasing it to the whole user base
Then conducting deep dives; quantitative user research and data analysis
Usually, users get to see a new feature only after it has passed A/B tests. Then we analyse the impact more broadly over time, which is what I’m doing at the moment.
It’s a foundational skill that any data scientist must master! It’s data science 101.
How to approach deep dives?
#1. Define the scope & objectives
What’s the core problem or question? For example:
What impact does feature X have on user engagement across different platforms?
Are there noticeable differences in user behaviour across different markets or product types due to feature X?
What do we aim to improve or solve with this analysis? Is it increasing user engagement? Improving user retention, or something else?
#2. Formulate hypotheses
Check past experiments and research to gather existing knowledge and data about this feature or similar others.
Based on that and your understanding of the subject, develop hypotheses. What are you expecting to find? This will guide your data collection & analysis. Be prepared to pivot if new, unexpected insights arise.
Then outline the types of data analysis that can be conducted:
Descriptive analytics to quantify reach and usage
Comparative analysis to identify behavioural differences across user groups
Regularly check your findings against your initial hypotheses & objectives. Adjust your approach based on what the data is telling you.
#3. Define the needed data
Identify what data points are necessary to test your hypotheses.
Start with a minimal dataset to validate your hypotheses, then expand as needed
Try to avoid spending weeks on something and then not meeting expectations
Begin with a clear and narrow scope. Limit the scope to manageable pieces that allow for incremental progress and adjustments based on findings.
#4. Anticipate actions
When drafting hypotheses and potential recommendations from these, think about whether those recommendations will indeed be impactful.
Short-term: How will these insights immediately inform strategy? For example, adjustments in marketing or product development
Long-term: How will these insights contribute to strategic planning or product roadmaps?
Identify which KPIs (Key Performance Indicators) or metrics are expected to be impacted by the findings. This may include engagement rates, conversion rates, etc.
Anticipate the actions product managers might take based on your findings to guide the analysis direction. What will they be inspired to take action on?
Depending on that, think of different trajectories. How do I want this to play out once it’s delivered?
#5. Define expected outputs
What will the output of this research look like?
A table summarizing the outcomes of the research, analysis, and past experiments?
Visualisations illustrating the potential impacts or trends observed?
What form might the recommendations take based on potential findings? These could range from strategic shifts to tactical adjustments in the product.
Prepare recommendations based on your findings. Each recommendation should be actionable and clearly tied to the insights derived from the data.
#6. Identify risks & limitations
What could potentially go wrong during this analysis? For example, biases in data, insufficient data coverage, or external factors might affect the analysis and results.
Clearly state the limitations of your data and methodology. This could include the scope of data, historical data availability, or the generalisability of the results
Outline steps or methods to mitigate these limitations, such as using robust statistical methods to handle biases
#7. Focus on storytelling & presentation
Think about the narrative of your presentation. How will you tell the story of your findings? The structure should logically flow as follows:
Introducing the problem → Presenting the data → Offering conclusions + recommendations
Make sure to:
Include an executive summary at the beginning of your report
Use visuals effectively to represent your data
Build each slide or section of your report upon the previous one to maintain a cohesive narrative
Finally, keep the feedback loop constantly going
While working through the results, make sure to regularly gather feedback from the product team. This is useful for making sure:
The analysis meets their expectations and the project stays on track
The insights are relevant and actionable from a product development perspective
Any ambiguity in what is expected from the analysis is clarified
In brief:
Start small: Tackle your analysis in manageable pieces.
Stay flexible: Be ready to adjust your hypotheses based on data insights.
Communicate clearly: Keep stakeholders informed throughout the process.
Use feedback constructively: Regularly incorporate feedback to refine your approach.
Try applying these tips in your next project and see the difference they make!
If you’re enjoying these dives into data science in the context of a top tech company, don’t forget to subscribe and follow along on YouTube, Instagram & LinkedIn for more updates and insights.
See you next week for more data stories 🫶